Semi-supervised learning in GANs can improve synthetic data generation by using a mix of labeled and unlabeled data to enhance the generator's ability to create realistic images while also respecting label constraints. Here are the key steps you can follow:
- Label Prediction: The discriminator is extended to predict the class label of both real and generated images using a softmax or similar classification method.
- Unlabeled Data: Both labeled and unlabeled images are used during training, with the discriminator being forced to classify the generated images correctly based on the constraints.
- Semi-supervised Loss: The loss includes both adversarial loss (for distinguishing real vs. fake) and classification loss (for label prediction).
Here is the code snippet you can refer to:
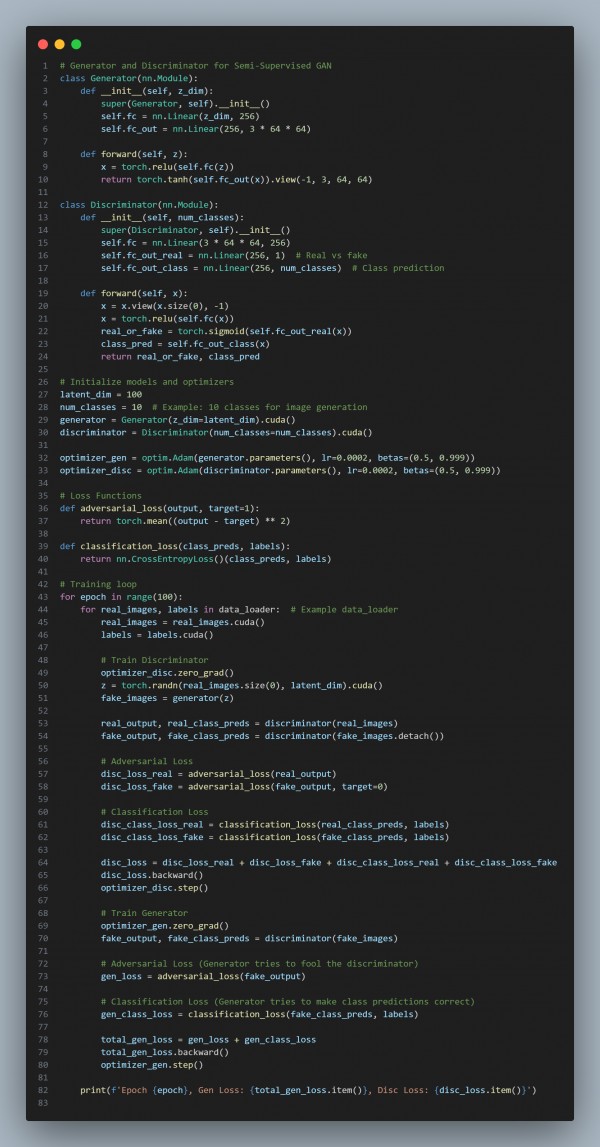
In the above code, we are using the following key features:
- Discriminator with Classification: The discriminator is tasked with both distinguishing real vs. fake images and predicting the class of the image.
- Semi-Supervised Learning: The discriminator is trained using both labeled and unlabeled data, making use of the class labels to improve training.
- Adversarial + Classification Loss: The generator is trained to minimize both adversarial loss (to generate realistic images) and classification loss (to respect the class labels).
- Improved Data Generation: The generator produces more class-specific realistic synthetic data, even with limited labeled data.
Hence, by referring to the above, you can improve synthetic data generation with label constraints.