Adversarial training can be used to reduce unrealistic image generation by employing the following strategies given below:
- Discriminator Feedback: The discriminator provides feedback to the generator, penalizing unrealistic or low-quality images. This forces the generator to produce more realistic images.
- Wasserstein Loss: Using a Wasserstein loss with gradient penalty in WGANs (Wasserstein GANs) can stabilize training and reduce mode collapse, ensuring more realistic and diverse images.
- Feature Matching: The discriminator can be used to match feature statistics between real and generated images, encouraging more realistic images by aligning their high-level features.
- Perceptual Loss: Incorporate perceptual loss based on pre-trained networks (e.g., VGG) to ensure the generated images align with real-world features, improving realism.
Here is the code snippet you can refer to:
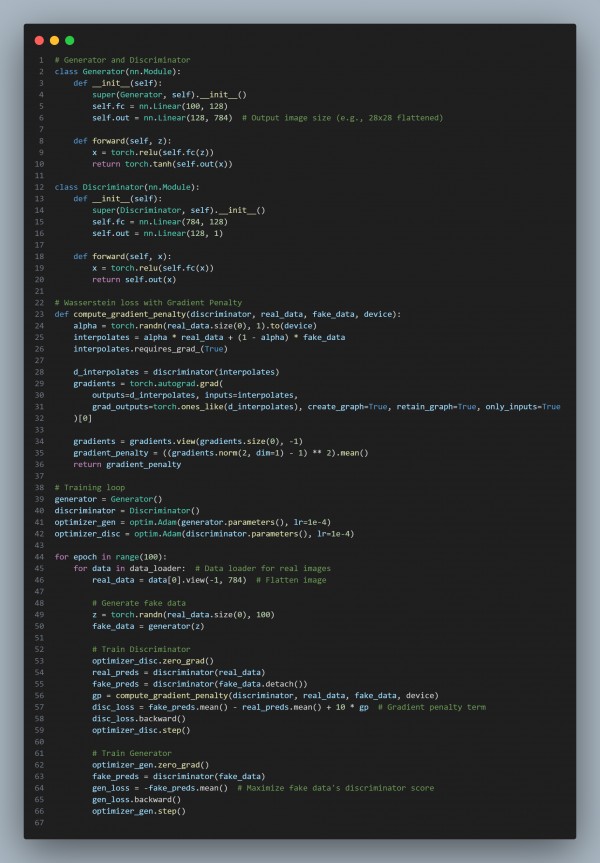
In the above code, we are using the following key strategies:
- Discriminator Feedback: Guides the generator to improve image quality by penalizing unrealistic outputs.
- Wasserstein Loss: Stabilizes training and reduces the risk of unrealistic image generation.
- Gradient Penalty: Smoothens training, preventing mode collapse and ensuring more realistic images.
- Feature Matching/Perceptual Loss: Ensures generated images are perceptually similar to real images.
Hence, by referring to the above, you can reduce unrealistic image generation in deep learning-based image models.