To manage catastrophic forgetting in continual learning for generative AI models, the following methods are effective:
- Elastic Weight Consolidation (EWC)
- Penalizes changes to weights critical to previous tasks.

- Replay Buffer
- Stores samples from previous tasks to retrain alongside new tasks.
- Generative Replay
- Uses a generative model to recreate data from earlier tasks for retraining.
\ 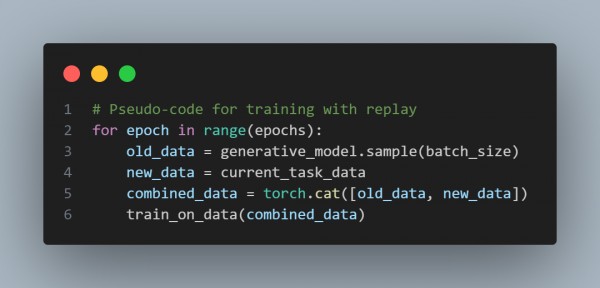
- Progressive Networks
- Adds new sub-networks for new tasks while freezing old ones.

- Knowledge Distillation
- Retains knowledge by minimizing divergence between old and new model outputs
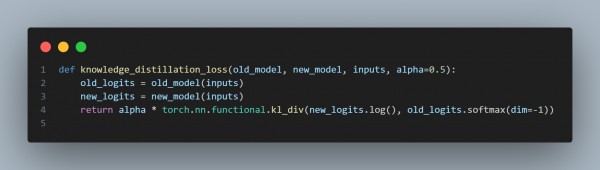
The above code uses methods to balance learning new tasks while retaining knowledge of previous tasks. A combination like EWC + Replay Buffer is often used for generative AI.
Hence, by referring to these methods, you can manage catastrophic forgetting in continual learning for generative AI models.