Latent space interpolation for style transfer in a GAN involves blending the latent vectors of two styles to generate new images that smoothly transition between the two styles. In order to implement, you can refer to the following code snippet below:
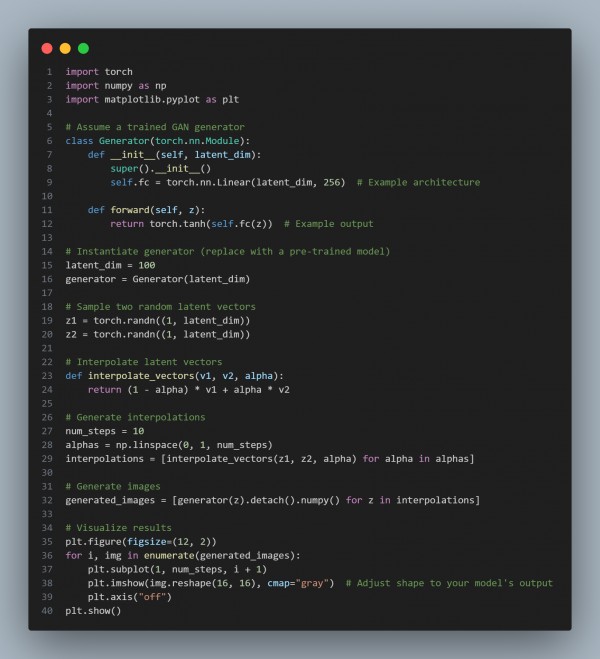
In the above code, we are using the following:
- Latent Vectors: z1 and z2 represent styles or features. Sampling from the GAN's latent space ensures the generator can produce meaningful outputs.
- Interpolation: The interpolate_vectors function computes a weighted average of the latent vectors controlled by the alpha parameter.
- Image Generation: The generator transforms each interpolated latent vector into an image.
- Visualization: The matplotlib library displays the smooth transition between styles.
Hence, this approach works for GANs like StyleGAN, where style transfer is highly effective due to well-structured latent spaces.