To implement domain adaptation in generative models for different datasets, you can use techniques like cycle consistency loss (e.g., CycleGAN) or domain adversarial training (e.g., DANN). These methods help the model generate samples that are consistent with a target domain while leveraging a source domain.
Here is the code snippet you can refer to:
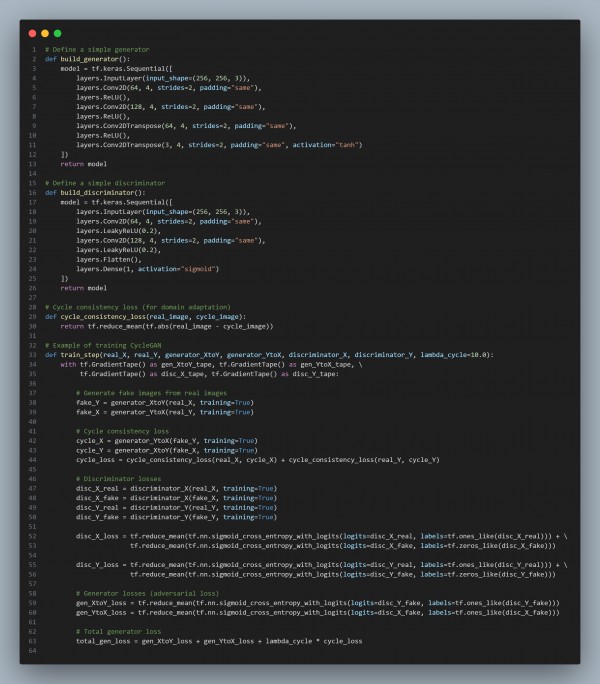
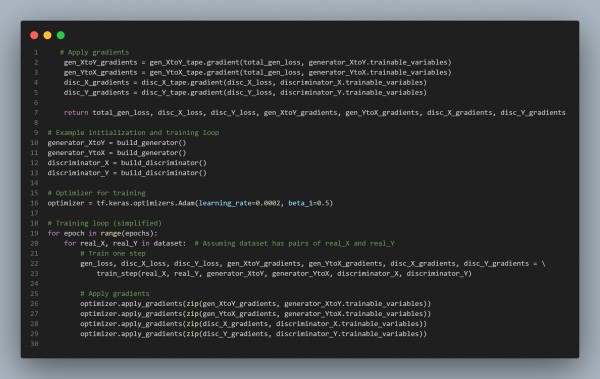
In the above code, we are using the following key strategies:
- CycleGAN: Use cycle consistency loss to ensure that generated images can be mapped back to the original domain.
- Domain Adversarial Networks (DANN): Use a domain classifier to encourage the model to generate domain-invariant features.
- Adversarial Training: Train the generator and discriminator adversarially to adapt to the target domain.
Hence, this approach helps the model adapt to new domains with minimal data by leveraging cross-domain image translation.