You can optimize training time in generative AI models using multiple dispatch by defining specialized functions for different types of data and models. Here is the code you can refer to:
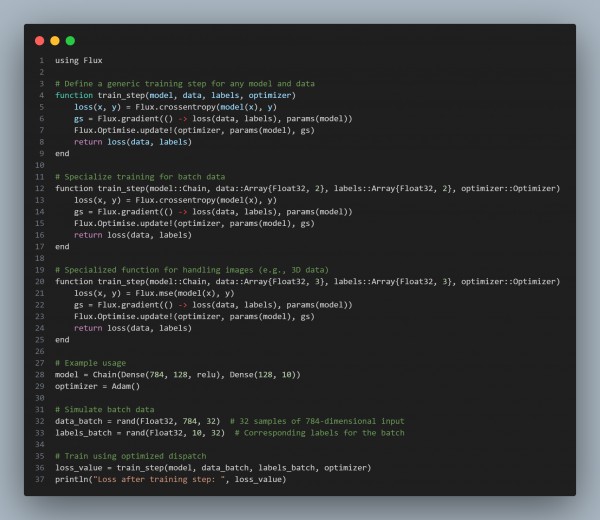
In the above code, we are using the following:
- Multiple Dispatch: Julia's multiple dispatch allows you to define different training steps for various data types (e.g., 2D for tabular data, 3D for images).
- Optimization: You can optimize operations for different data shapes, leading to better performance by reducing unnecessary computations or adapting the model's operations to the specific structure of the input data.
- Efficiency: Specialized functions reduce overhead and increase efficiency by directly mapping the operations to the data type (e.g., vectorized operations for batch data or image-specific operations).
Hence, by leveraging Julia's multiple dispatch, you can efficiently optimize training steps, especially in generative AI tasks where data types and model architectures vary widely.